Dual Accuracy-Quality-Driven Neural Network for Prediction Interval Generation
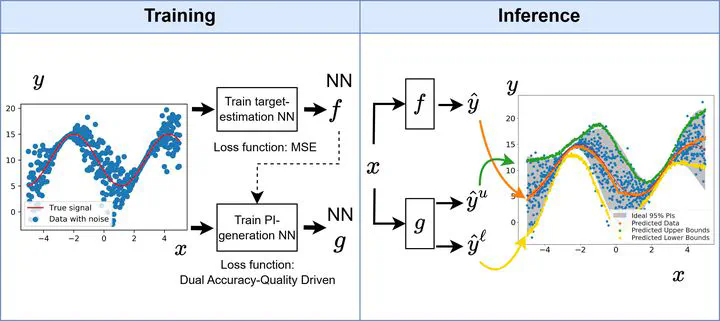
Abstract
Accurate uncertainty quantification is necessary to enhance the reliability of deep learning (DL) models in real-world applications. In the case of regression tasks, prediction intervals (PIs) should be provided along with the deterministic predictions of DL models. Such PIs are useful or “high-quality (HQ)” as long as they are sufficiently narrow and capture most of the probability density. In this article, we present a method to learn PIs for regression-based neural networks (NNs) automatically in addition to the conventional target predictions. In particular, we train two companion NNs: one that uses one output, the target estimate, and another that uses two outputs, the upper and lower bounds of the corresponding PI. Our main contribution is the design of a novel loss function for the PI-generation network that takes into account the output of the target-estimation network and has two optimization objectives: minimizing the mean PI width and ensuring the PI integrity using constraints that maximize the PI probability coverage implicitly. Furthermore, we introduce a self-adaptive coefficient that balances both objectives within the loss function, which alleviates the task of fine-tuning. Experiments using a synthetic dataset, eight benchmark datasets, and a real-world crop yield prediction dataset showed that our method was able to maintain a nominal probability coverage and produce significantly narrower PIs without detriment to its target estimation accuracy when compared to those PIs generated by three state-of-the-art neural-network-based methods. In other words, our method was shown to produce higher quality PIs.
Type
Publication
IEEE Transactions on Neural Networks and Learning Systems